-
shima__shima
- 8267
- 24
- 3
- 0

So proud of @_leotrs, who presented at his 1st KDD tutorial on Graph Metric Spaces: neu-spiral.github.io/GraphMetricSpa… #KDD2018 pic.twitter.com/6u8n2lvNIt
2018-08-19 20:12:08

Great tutorial at #KDD2018 #datascience in #Retail as-a-Service aigsic.com/kdd_2018_raas.… pic.twitter.com/TnZtYBxNqh
2018-08-19 20:58:28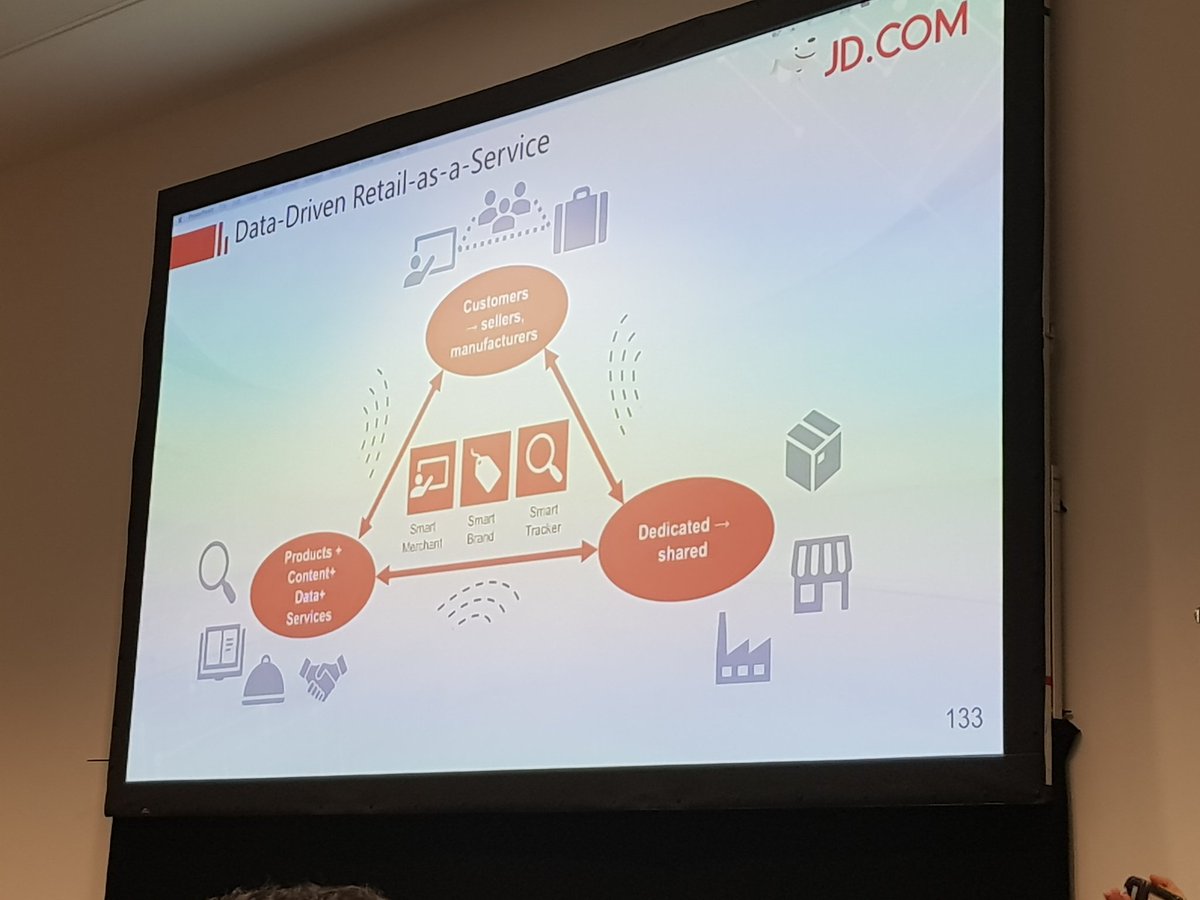

Fact Checking: Theory and Practice #KDD2018 Tutorial Slides shiralkarprashant.github.io/fact-checking-… pic.twitter.com/sDNlYiIXAT
2018-08-19 21:07:18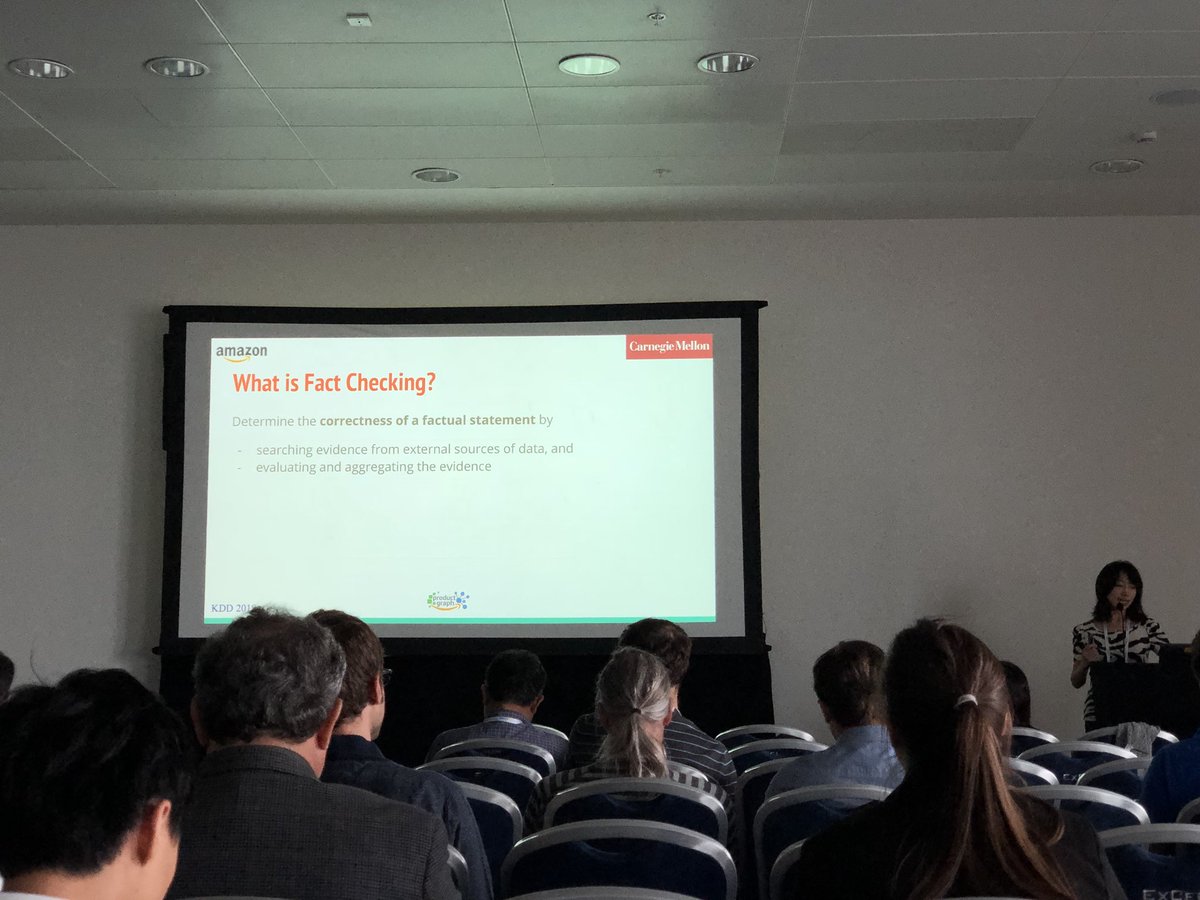

My first conf talk ever was at #KDD2001 using slides and analog animations on an overhead projector. Now, I, am back at #KDD2018. Exciting program so far! The content shifted quite a bit since 2001 from *-mining to *-data science.
2018-08-19 21:08:59
#kdd2018 tutorial on “Causal Inference & Counterfactual Reasoning” starting now by @amt_shrma & @emrek — From data to predictions — From prediction to decision making — Interventions change the environment pic.twitter.com/jjuMYU5V53
2018-08-19 21:13:04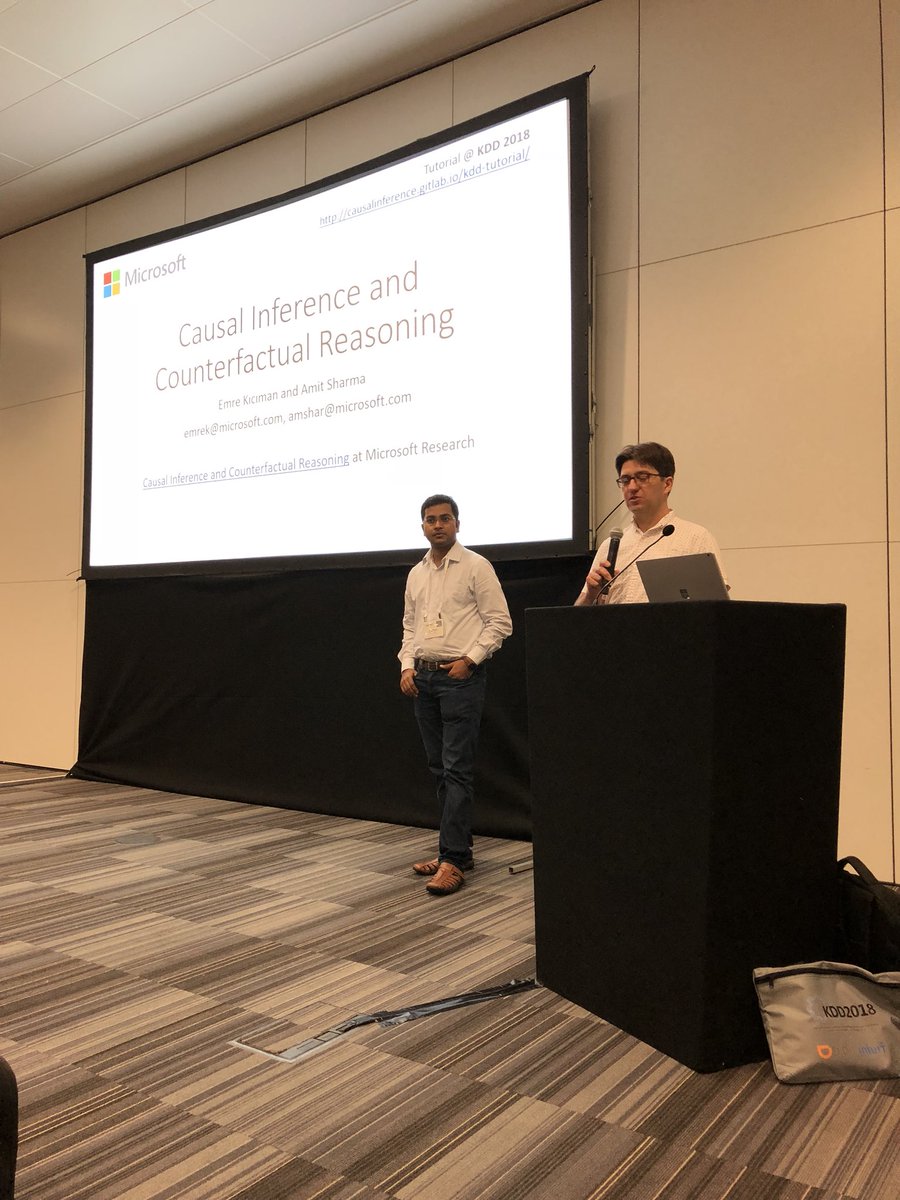
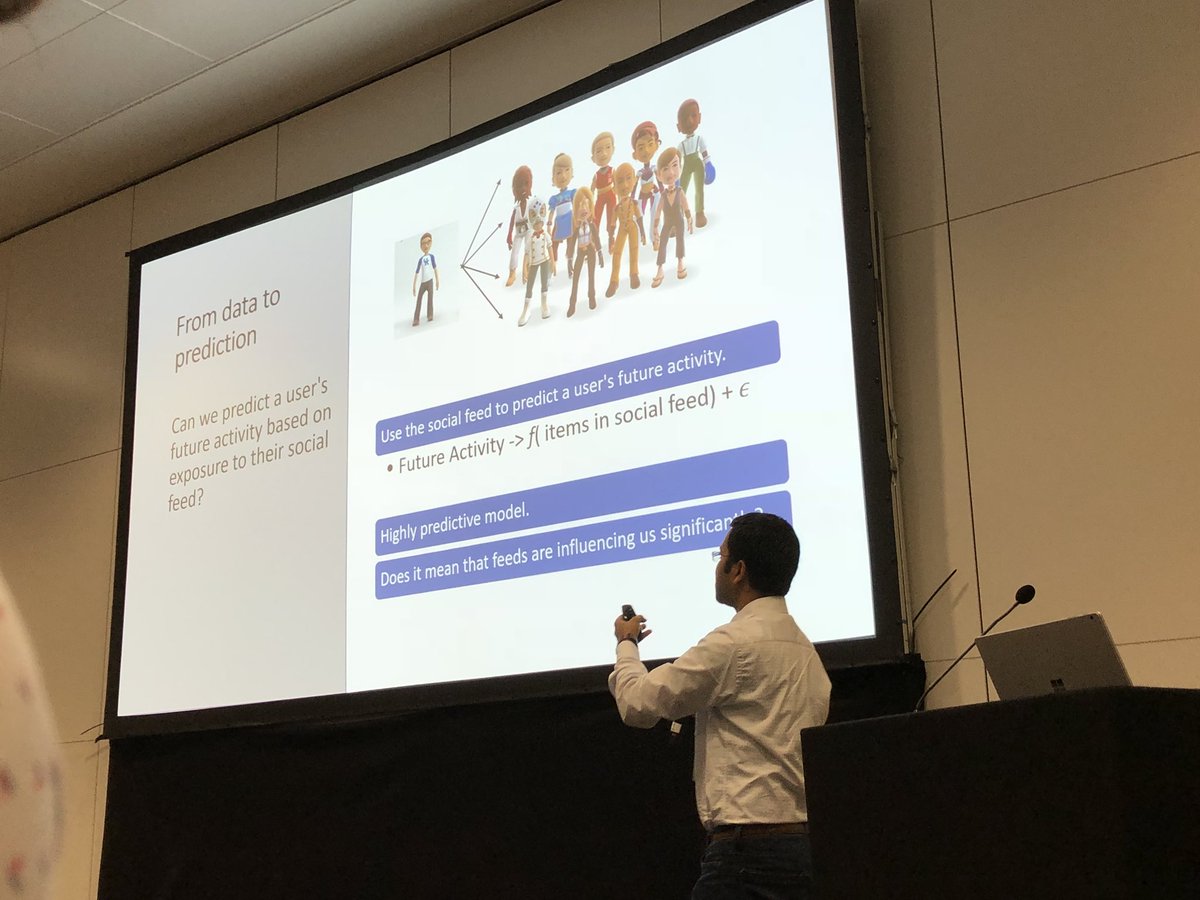
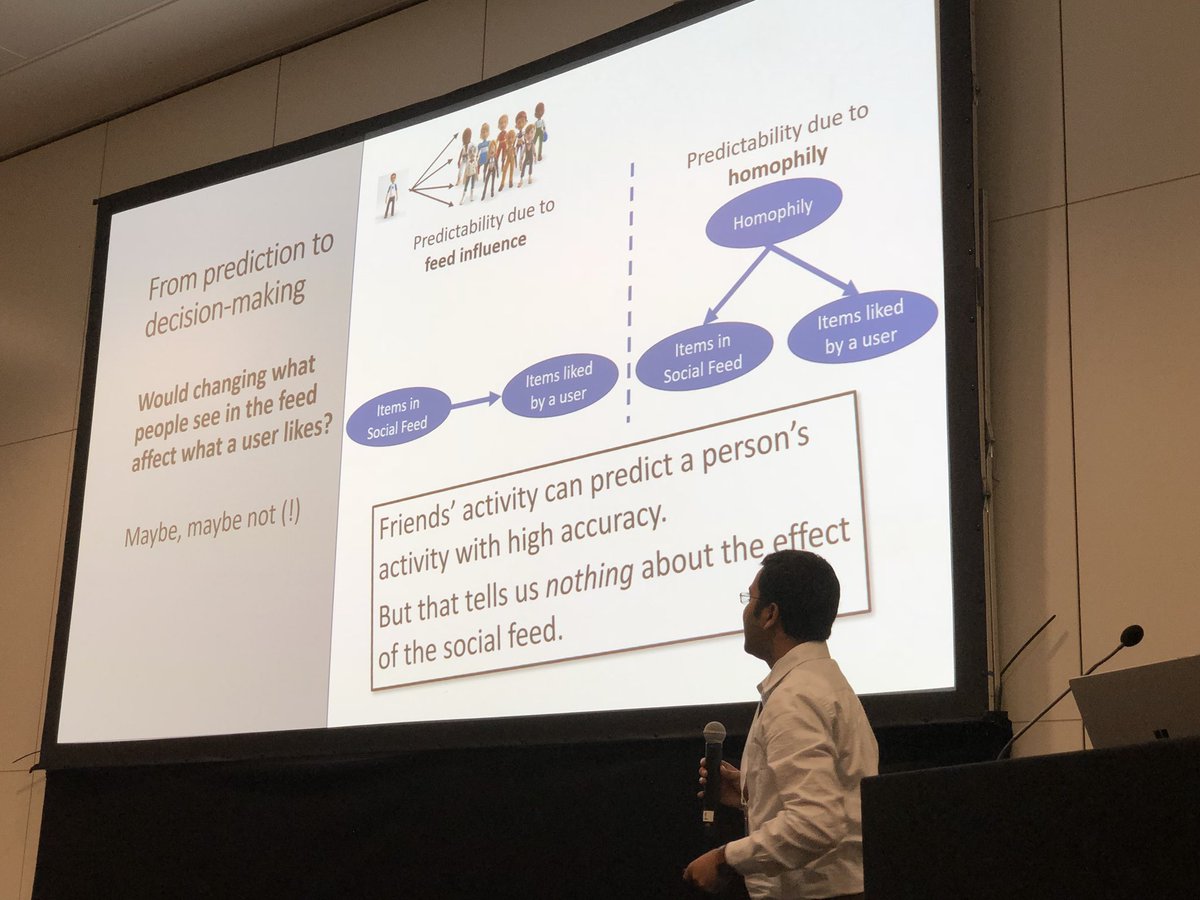
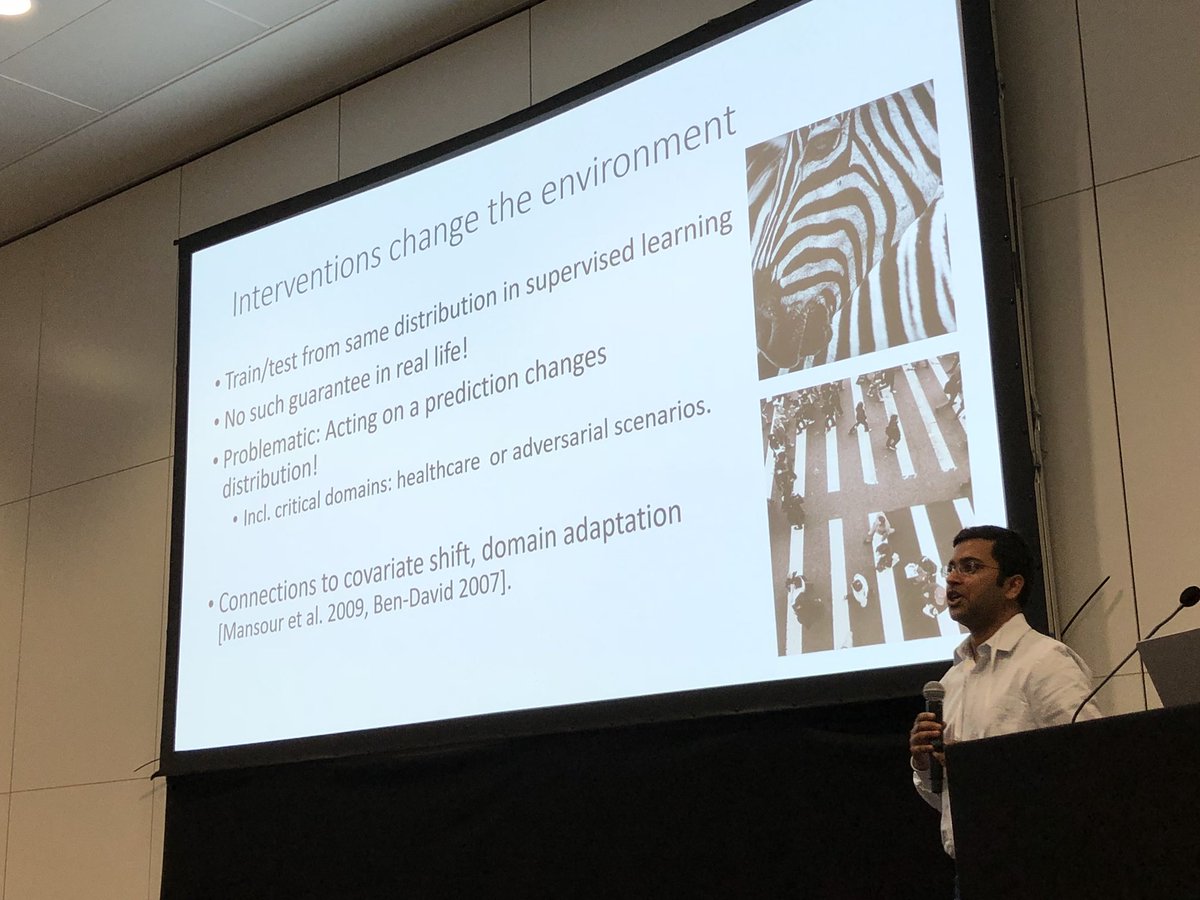

predictive models are insufficient & hence we need causal models to reason with data #kdd2018 causal inference tutorial pic.twitter.com/BXhbD9ghhu
2018-08-19 21:15:06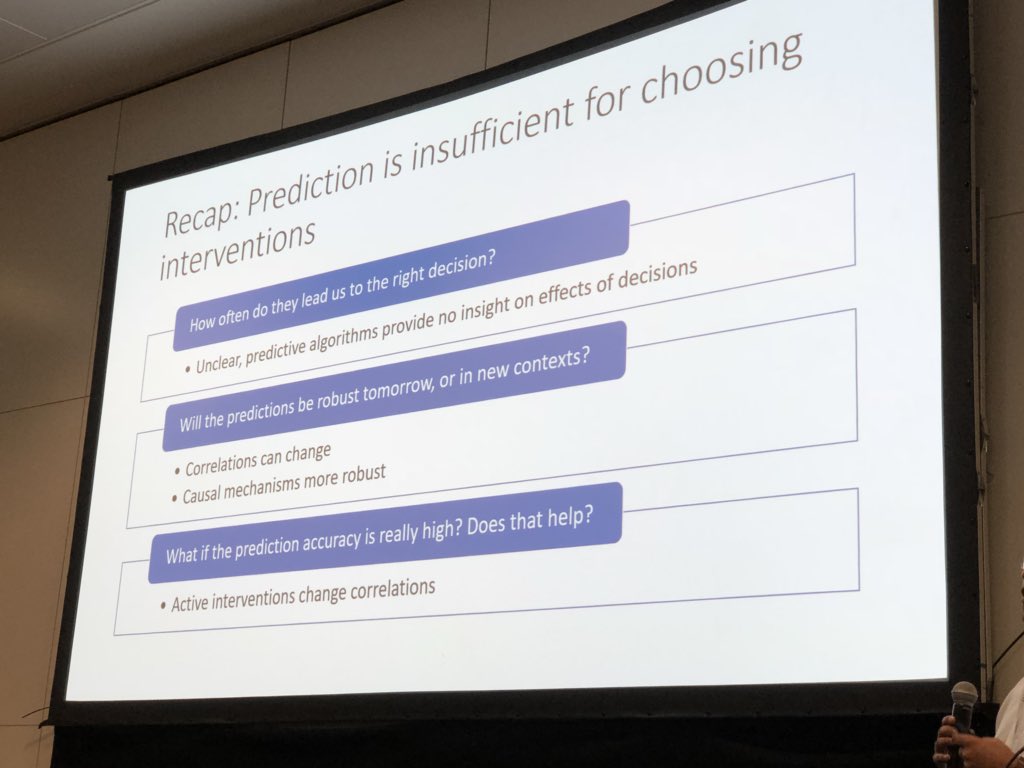

Tanya Berger-Wolf diving deep into which network representation can best answer questions on you data in Modeling Data with Networks tutorial (room 11). #KDD2018 pic.twitter.com/Fan6cOmPMY
2018-08-19 21:15:28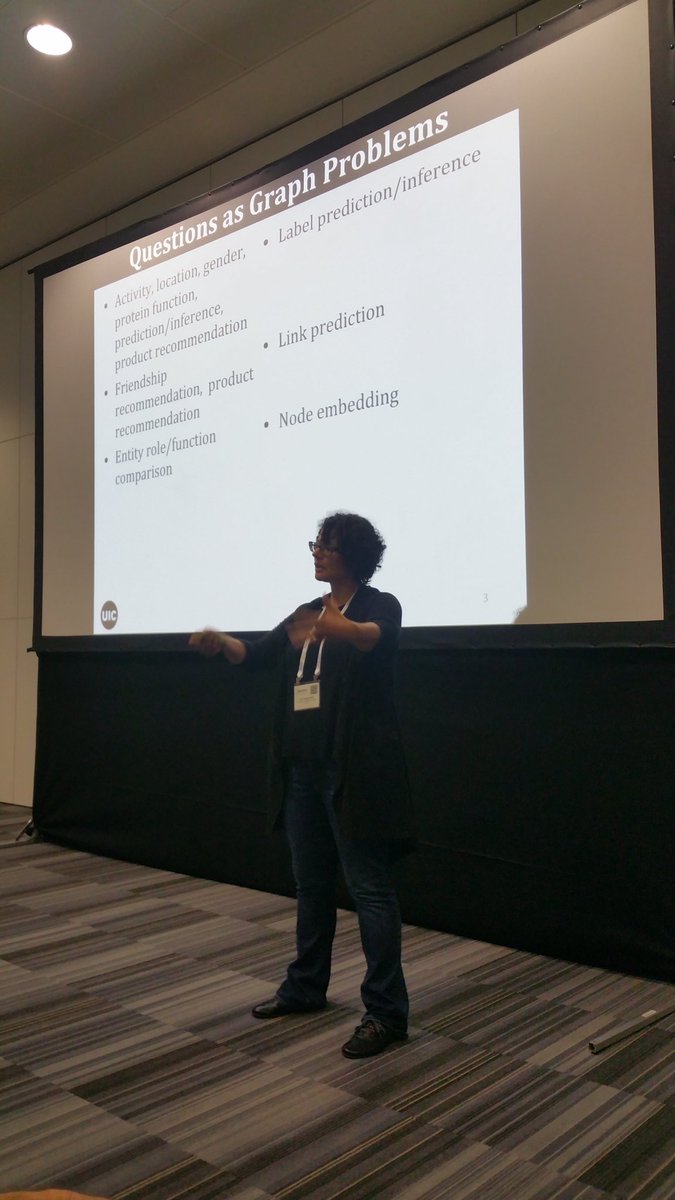

Full room for causal inference tutorial by #Microsoft research #KDD2018 pic.twitter.com/xCeiSWGQvh
2018-08-19 21:17:20

Judea Pearl’s 3 layer Causal Hierarchy - association - interventions - counterfactuals #kdd2018 tutorial om causal inferences pic.twitter.com/QiRkl40r2e
2018-08-19 21:19:22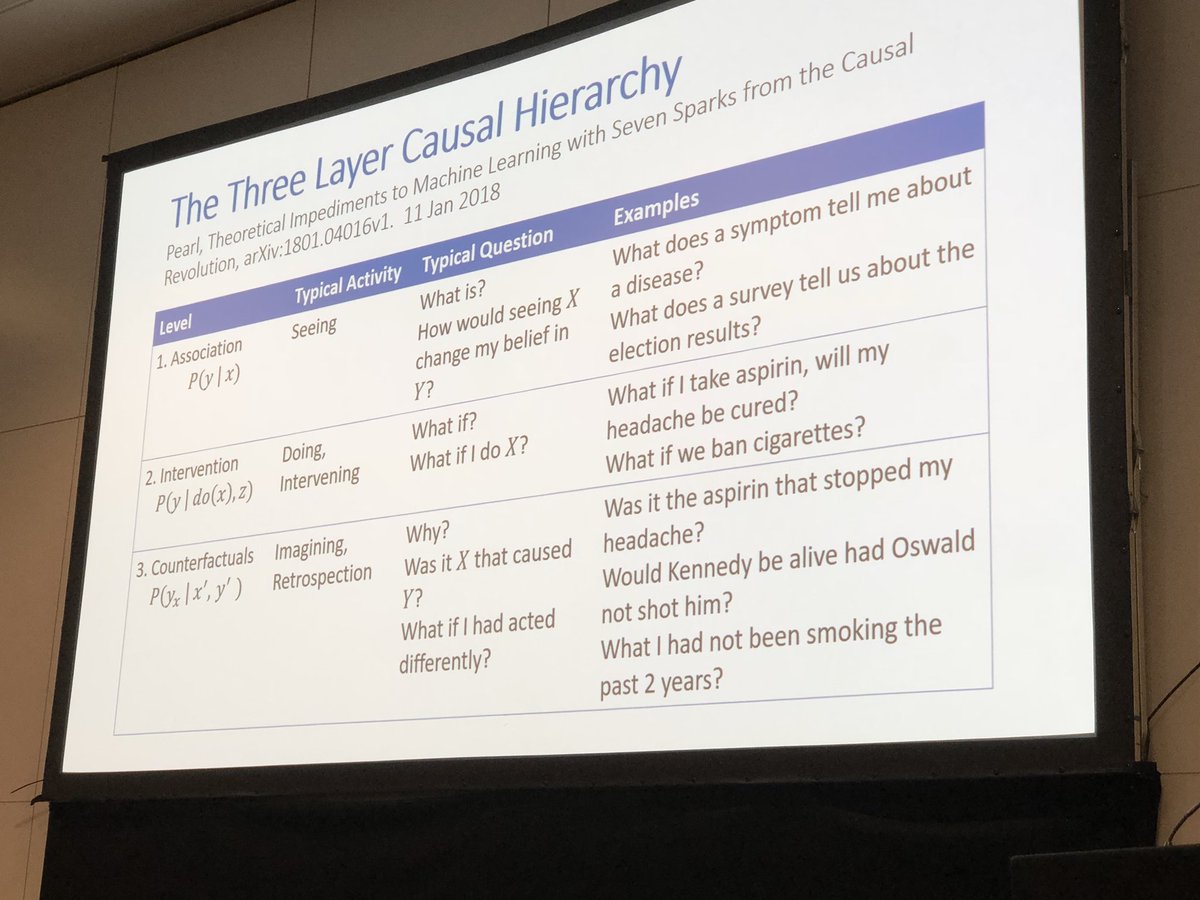

The slides for our Tutorial are available on the tutorial page mlhealthcare.github.io #KDD2018 #KDD
2018-08-19 21:23:12
Looking forward to 3 exciting days at #KDD2018. #datamining #informationnetworks #AI #Deeplearning pic.twitter.com/7nHuThVo5q
2018-08-19 21:26:55

Packed room for @emrek's and Amit Sharma's tutorial on causal reasoning at #KDD2018. @MSFTResearch pic.twitter.com/RUsMeaVpy4
2018-08-19 21:50:49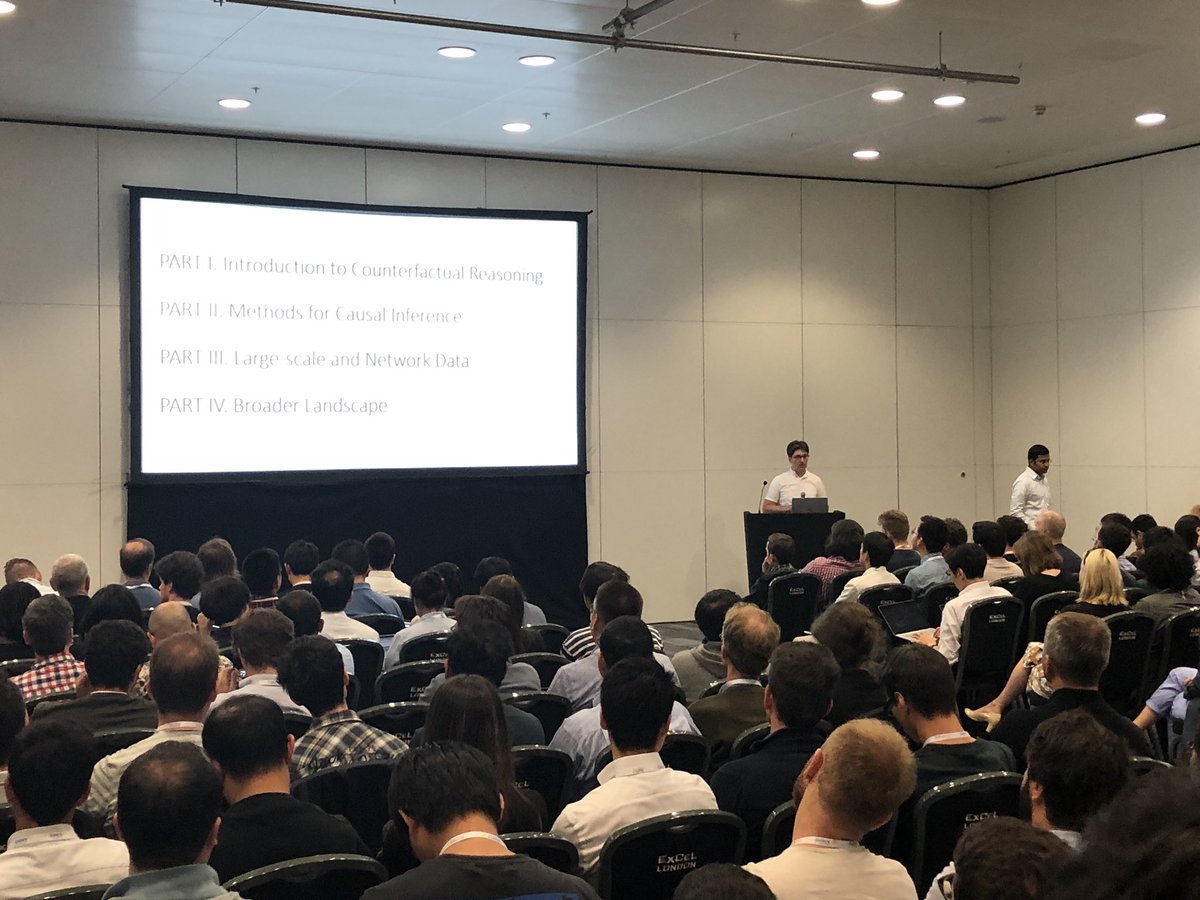

Explainability of ML in healthcare #KDD2018 pic.twitter.com/N3S2TrOn7k
2018-08-19 21:53:36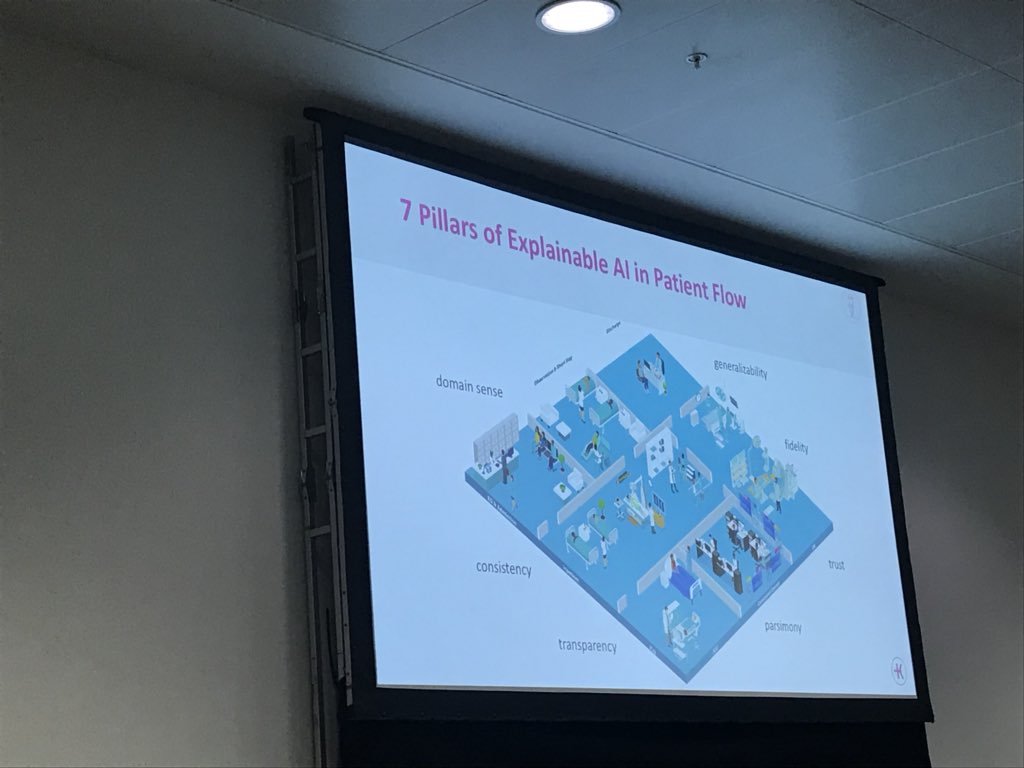

80% of data is unstructured Data. Labeling and topic modelling are critical for high quality analyses. #KDD2018 pic.twitter.com/vm9wQr6W1J
2018-08-19 22:05:36

Frameworks to make causal estimates: — Potential outcomes framework — Structured Causal Models (SCM) SCM allows us to leverage Pearl’s causality to express counterfactuals (using do-calculus) impressive overview of the field by @amt_shrma @emrek #kdd2018 causality tutorial pic.twitter.com/dmNYdi2YWI
2018-08-19 22:14:23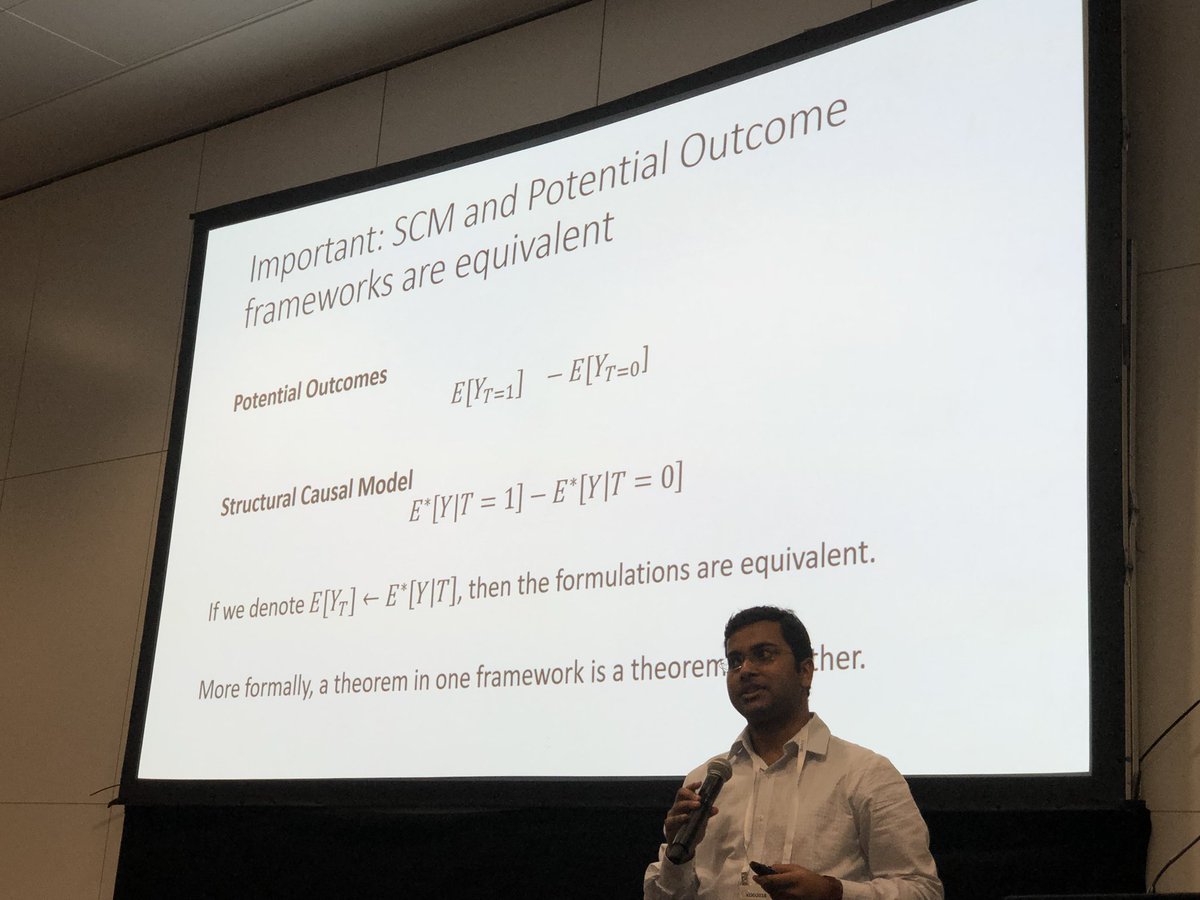
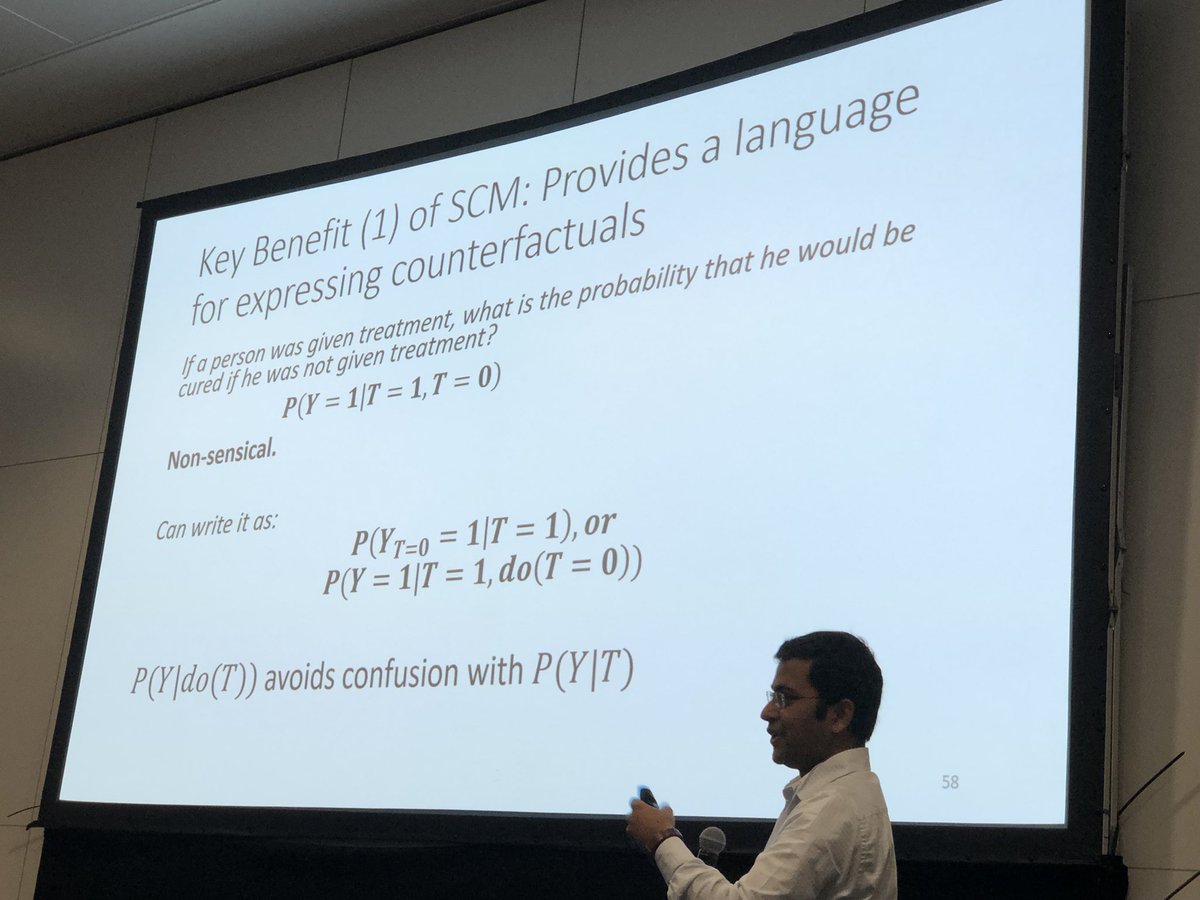
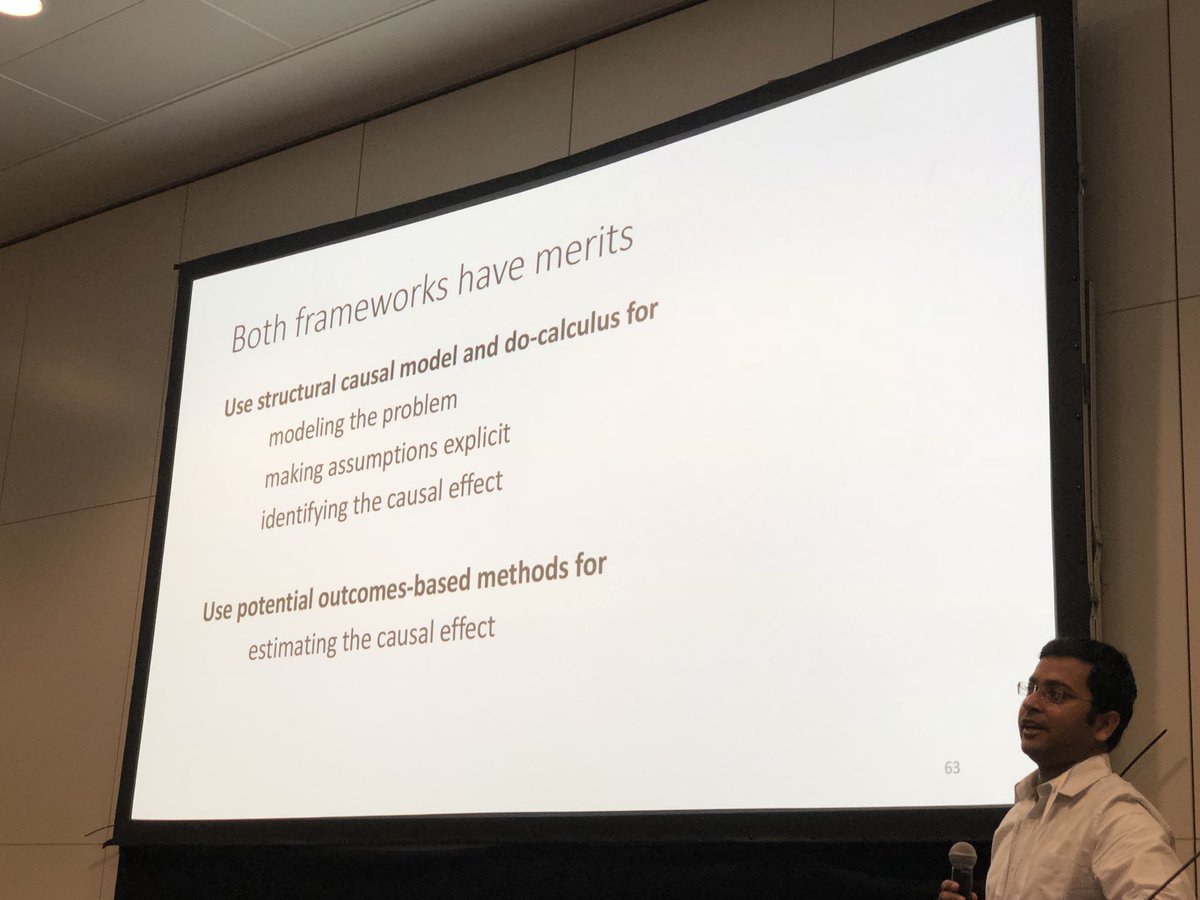

New meaning to my name 'ELSA' 😀#KDD2018 #London pic.twitter.com/wUVKYtNRvL
2018-08-19 22:14:47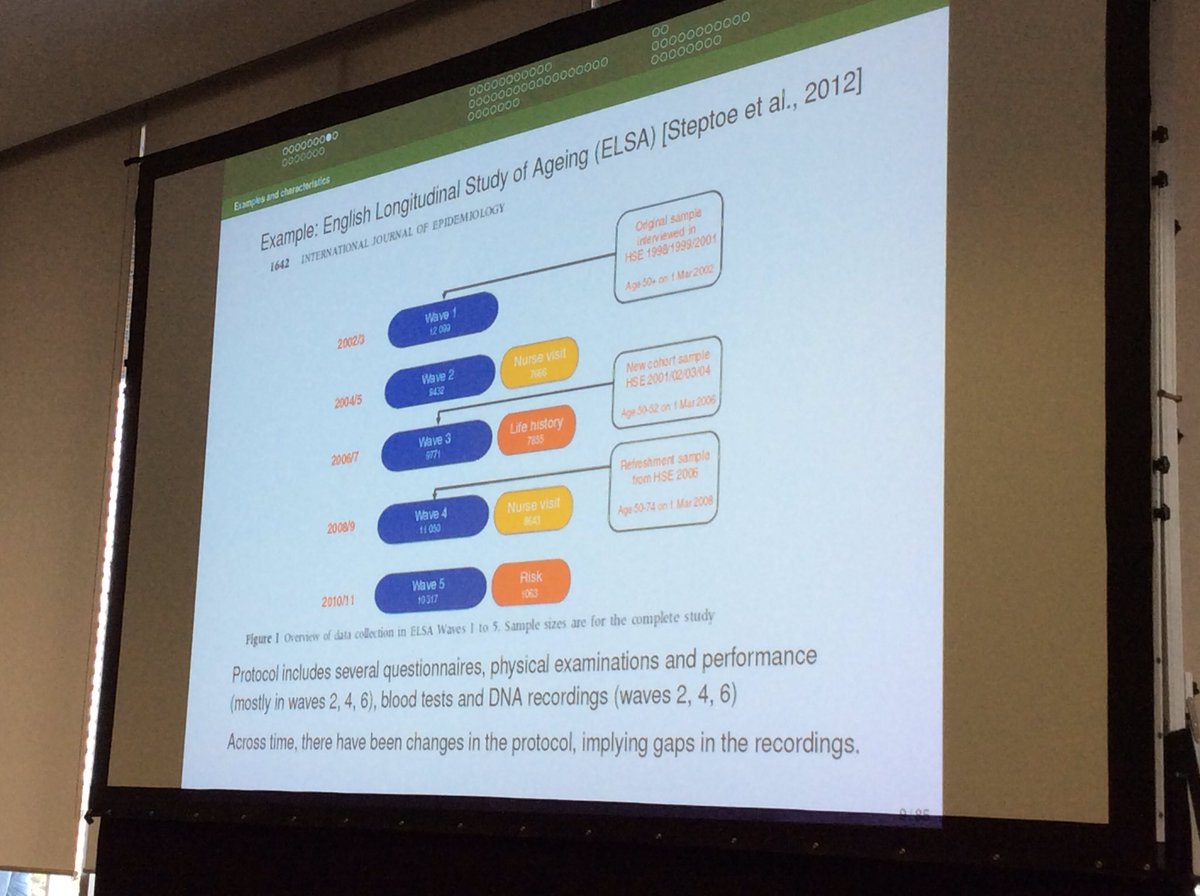

Fact Checking using Knowledge Graphs #KDD2018 pic.twitter.com/MUowj93nSd
2018-08-19 22:19:04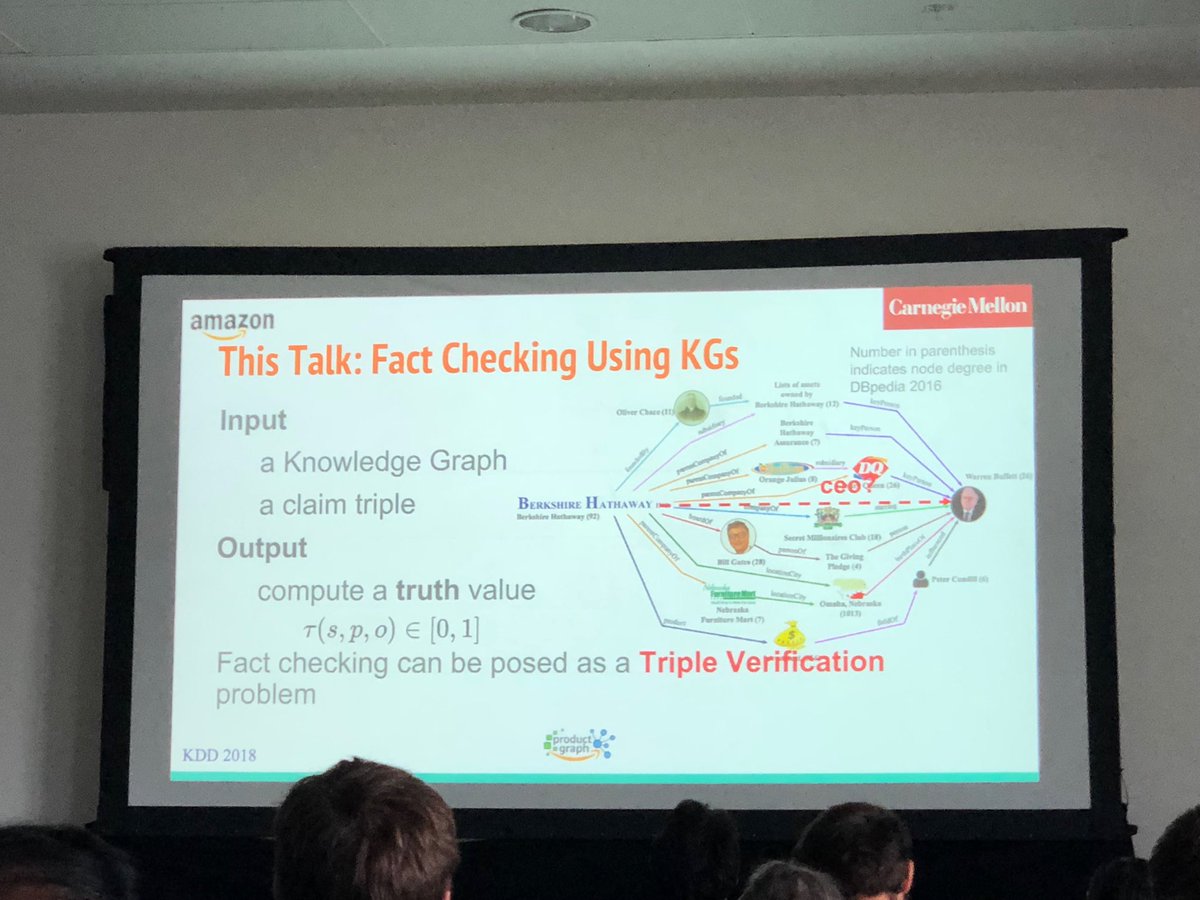

Goals of network embedding - support structural properties for inference - @jian_pei #KDD2018 pic.twitter.com/71Wnwvfknb
2018-08-19 22:19:41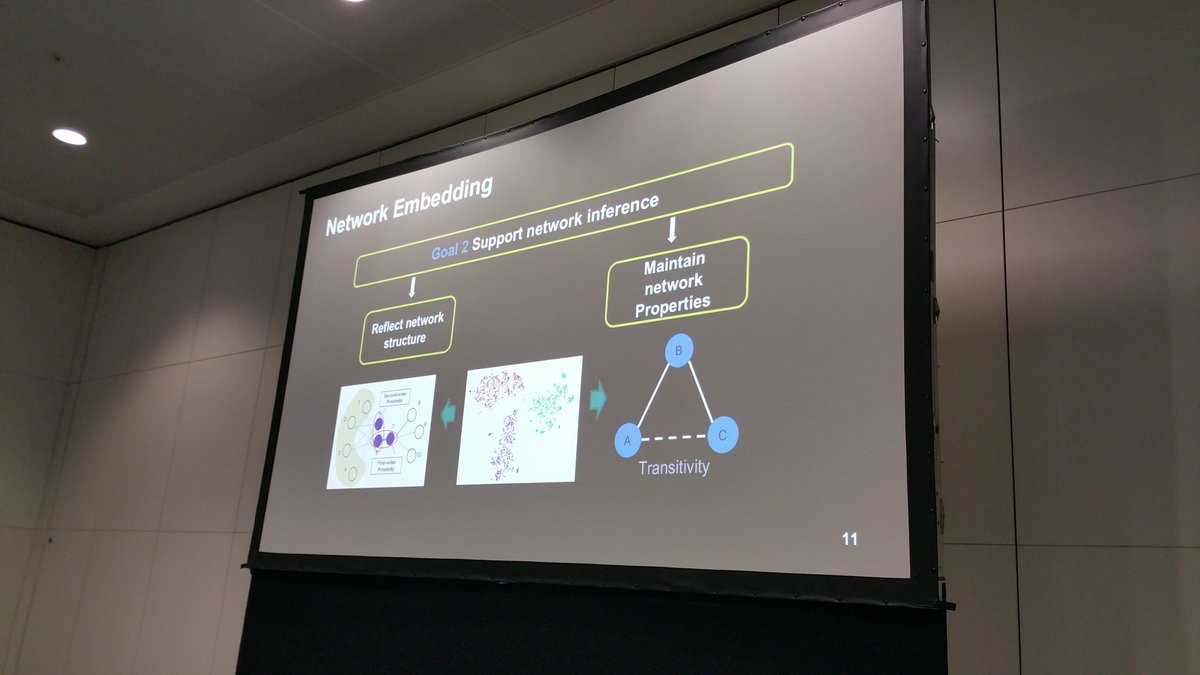

#kdd2018 #datascience 🤗🤗 @ London, United Kingdom instagram.com/p/BmqZ8p4Fb23/…
2018-08-19 22:44:12
私の調査が手薄な方面.因果グラフの存在を存在を前提にしてるので,細かい分析はできる.因果グラフをデータから求める研究のと,因果分析を同時にするという立場で機械学習の公平性を見るという風にもできるのかな?
2018-08-19 22:50:13
午後はfactchecking のチュートリアル shiralkarprashant.github.io/fact-checking-… あまりKDDでも見ない話題,CMU の Faloutsos とAmazonの中の人による講演
2018-08-19 22:50:24
fact checking:外部ソースからエビデンスを集め,その信頼性を評価して集約する. こうなるとQAとかと似てる気がする.
2018-08-19 22:50:39
信頼性推定は,情報から情報源の信頼性を求め,信頼できる情報源の情報を集約するとかいう,クラウドソーシングのdavid-sekine みたいな感じになってて,既存技術は使えそう.一方で,コピペ関係の情報源を特定して,情報の信頼性を調整するとか,独自のものもあるようだ.
2018-08-19 22:50:51
また知識グラフはより積極的に利用されてる感じ.事実の検証は,セマンティックWebの最上位層のlogicが担うことを期待されていたが,別の手段により知識グラフは検証作業に使われているみたいだ.
2018-08-19 22:51:03